


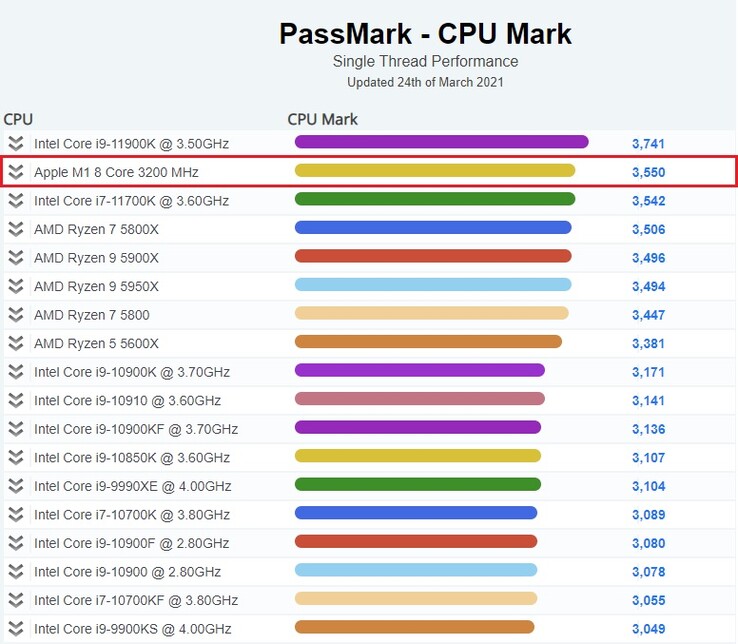
But even state-of-the-art neural network models can be non-generalizable in specific studies due to the large-scale demand for data ( Karpatne et al., 2017 Zhao et al., 2022). In many respects, the prediction accuracy of this method far exceeds that of traditional modeling methods due to the coverage of the vast training dataset ( Valsamis et al., 2017 Sun et al., 2022 Wu et al., 2022 Yun and). This saves us from knowing the specific equations between the data, and the algorithm will automatically derive the data to match the target value. The introduction of ML and deep learning methods achieved a leap from “model-driven” to “data-driven” ( Xue et al., 2015 Jiang et al., 2021 Liu et al., 2022). Machine learning (ML) methods based on neural network models automatically extract complex relationships from data, theoretically solving scientific problems in physical models ( Bentley, 2014 Kelley et al., 2016 Biswas et al., 2018 Huang et al., 2021 Jiang et al., 2021). In addition, the physical model contains many parameters estimated from limited observational data, which further degrades the performance of the physical model ( Günther et al., 2020). However, the deviation between the actual engineering process and the physical laws adopted in the physical model makes us need to approximate the real value, leading to errors ( Yan et al., 2020). In recent years, physics-based modeling approaches have become widely used analytical tools in engineering and environmental systems ( Willard et al., 2022). This paper combines various machine learning algorithms and computational fluid dynamics (CFD) simulation methods to systematically analyze the immune cell infiltration mechanism associated with liver cancer from the perspectives of biomedicine and mechanics. Previous studies have primarily interpreted the infiltration of immune cells from a biomedical perspective, ignoring the physical role in immune regulation. Among them, numerous microvascular stenosis fragments smaller than the cell size and tiny pores on the vessel wall are the key factors hindering the infiltration of immune cells. A key issue in immune infiltration is the transendothelial transmigration of leukocytes (immune cells). The mechanism of immune infiltration involving immune cells is closely related to various diseases. Compared with traditional CFD simulations, BPNN models avoid complex and time-dependent physical modeling and computational processes. The two prediction models achieved the prediction of leukocyte transmigration in a specific microvascular environment and maintained a high prediction accuracy, indicating the feasibility and robustness of the BPNN model applied to the prediction of immune cell infiltration. In the test data set, it has high prediction accuracy for cell deformation, motion velocity and flow lift forces during downstream motion, and maintains a good prediction accuracy for drag force. The model accurately predicted the cell movement and flow field changes during the transmigration. The model can efficiently predict the immune cell transmigration in a special microvascular environment, and obtain good prediction accuracy. By integrating the immune cell transmigration computational fluid dynamics (CFD) data, the paper builds a time-dependent leukocyte transmigration prediction model based on the bio-inspired methods, namely back propagation neural networks (BPNN) model. The physical mechanism of leukocyte infiltration remains to be explored. Previous studies have primarily interpreted the leukocyte infiltration of from biomedical perspective. A key issue in immune infiltration is the transendothelial transmigration of leukocytes. 2State Key Laboratory of Trauma, Burns and Combined Injury, Department of Wound Infection and Drug, Daping Hospital, Army Medical University, Chongqing, China.1Department of Engineering Structure and Mechanics, School of Science, Wuhan University of Technology, Wuhan, China.Qingjia Chi 1 Zichang Yang 1 Hua-Ping Liang 2*
